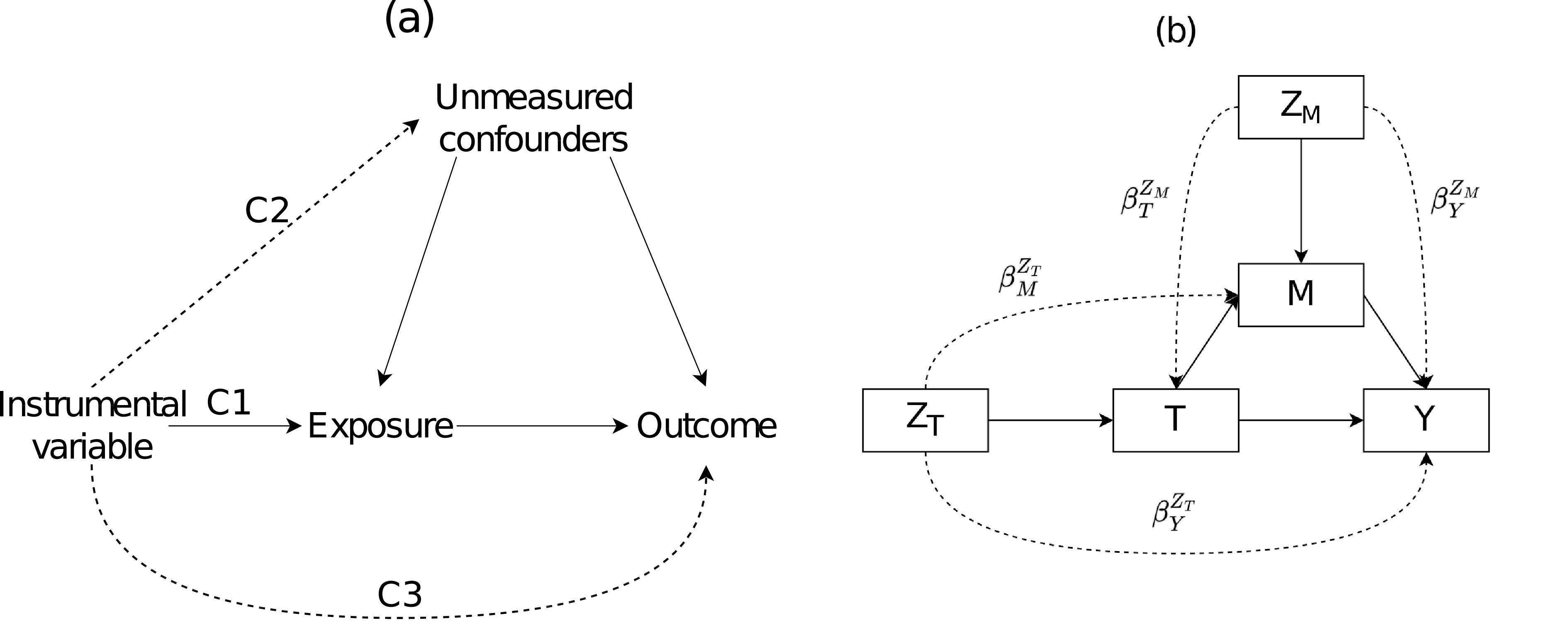
Mendelian randomization (MR) is widely used in causal mediation analysis to control unmeasured confounding effects, which is valid under some strong assumptions. It is thus of great interest to assess the impact of violations of these MR assumptions through sensitivity analysis. Sensitivity analyses have been conducted for simple MR-based causal average effect analyses, but they are not available for MR-based mediation analysis studies, and we aim to fill this gap in this paper. We propose to use two sensitivity parameters to quantify the effect due to the deviation of the IV assumptions. With these two sensitivity parameters, we derive consistent indirect causal effect estimators and establish their asymptotic propersties. Our theoretical results can be used in MR-based mediation analysis to study the impact of violations of MR assumptions. The finite sample performance of the proposed method is illustrated through simulation studies, sensitivity analysis, and application to a real genome-wide association study.
A MR-based mediation model with possibly invalid IVs.
Figure
1.
(a) The DAG of a simple MR model with a possibly invalid IV. C1 is the relevance assumption; C2 is the exchangeability assumption; C3 is the exclusion restriction. (b) The DAG of a mediation model with two IVs
[1] |
Smith G D, Ebrahim S. Mendelian randomization: prospects, potentials, and limitations. International Journal of Epidemiology, 2004, 33 (1): 30–42. DOI: 10.1093/ije/dyh132
|
[2] |
Katan M B. Apolipoprotein E isoforms, serum cholesterol, and cancer. The Lancet, 1986, 327 (8479): 507–508. DOI: 10.1016/s0140-6736(86)92972-7
|
[3] |
Burgess S, Scott R A, Timpson N J, et al. Using published data in Mendelian randomization: a blueprint for efficient identification of causal risk factors. European Journal of Epidemiology, 2015, 30 (7): 543–552. DOI: 10.1007/s10654-015-0011-z
|
[4] |
Relton C L, Smith G D. Two-step epigenetic Mendelian randomization: a strategy for establishing the causal role of epigenetic processes in pathways to disease. International Journal of Epidemiology, 2012, 41 (1): 161–176. DOI: 10.1093/ije/dyr233
|
[5] |
Angrist J D, Imbens G W, Rubin D B. Identification of causal effects using instrumental variables. Journal of the American Statistical Association, 1996, 91 (434): 444–455. DOI: 10.1080/01621459.1996.10476902
|
[6] |
Burgess S, Daniel R M, Butterworth A S, et al. Network Mendelian randomization: using genetic variants as instrumental variables to investigate mediation in causal pathways. International Journal of Epidemiology, 2015, 44 (2): 484–495. DOI: 10.1093/ije/dyu176
|
[7] |
Burgess S, Thompson S G. Multivariable Mendelian randomization: The use of pleiotropic genetic variants to estimate causal effects. American Journal of Epidemiology, 2015, 181 (4): 251–260. DOI: 10.1093/aje/kwu283
|
[8] |
The Global Burden of Metabolic Risk Factors for Chronic Diseases Collaboration (BMI Mediated Effects). Metabolic mediators of the effects of body-mass index, overweight, and obesity on coronary heart disease and stroke: A pooled analysis of 97 prospective cohorts with 1.8 million participants. The Lancet, 2014, 383 (9921): 970–983. DOI: 10.1016/S0140-6736(13)61836-X
|
[9] |
Carter A R, Gill D, Davies N M, et al. Understanding the consequences of education inequality on cardiovascular disease: Mendelian randomisation study. BMJ, 2019, 365: l1855. DOI: 10.1136/bmj.l1855
|
[10] |
Gill D, Cameron A C, Burgess S, et al. Urate, blood pressure, and cardiovascular disease: Evidence from Mendelian randomization and meta-analysis of clinical trials. Hypertension, 2021, 77 (2): 383–392. DOI: 10.1161/HYPERTENSIONAHA.120.16547
|
[11] |
Sanderson E. Multivariable Mendelian randomization and mediation. Cold Spring Harbor Perspectives in Medicine, 2021, 11 (2): a038984. DOI: 10.1101/cshperspect.a038984
|
[12] |
Morgan S, Winship C. Counterfactual and Causal Inference: Methods and Principles for Social Research. Cambridge, UK: Cambridge University Press, 2007.
|
[13] |
Small D S. Sensitivity analysis for instrumental variables regression with overidentifying restrictions. Journal of the American Statistical Association, 2007, 102 (479): 1049–1058. DOI: 10.1198/016214507000000608
|
[14] |
Zhang W, Ghosh D. A general approach to sensitivity analysis for Mendelian randomization. Statistics in Biosciences, 2021, 13 (1): 34–55. DOI: 10.1007/s12561-020-09280-5
|
[15] |
Wang X, Jiang Y, Zhang N R, et al. Sensitivity analysis and power for instrumental variable studies. Biometrics, 2018, 74 (4): 1150–1160. DOI: 10.1111/biom.12873
|
[16] |
Carter A R, Sanderson E, Hammerton G, et al. Mendelian randomisation for mediation analysis: current methods and challenges for implementation. European Journal of Epidemiology, 2021, 36 (5): 465–478. DOI: 10.1007/s10654-021-00757-1
|
[17] |
Baron R M, Kenny D A. The moderator-mediator variable distinction in social psychological research: conceptual, strategic, and statistical considerations. Journal of Personality and Social Psychology, 1986, 51 (6): 1173–1182. DOI: 10.1037//0022-3514.51.6.1173
|
[18] |
MacKinnon D P. Introduction to Statistical Mediation Analysis. New York: Routledge, 2008.
|
[19] |
Basmann R L. A generalized classical method of linear estimation of coefficients in a structural equation. Econometrica, 1957, 25: 77–83. DOI: 10.2307/1907743
|
[20] |
Burgess S, Thompson S G, CRP CHD Genetics Collaboration. Avoiding bias from weak instruments in Mendelian randomization studies. International Journal of Epidemiology, 2011, 40 (3): 755–764. DOI: 10.1093/ije/dyr036
|
[21] |
MacKinnon D P, Lockwood C M, Hoffman J M, et al. A comparison of methods to test mediation and other intervening variable effects. Psychological Methods, 2002, 7 (1): 83–104. DOI: 10.1037/1082-989x.7.1.83
|
[22] |
Zhao J, Bradfield J P, Li M, et al. The role of obesity-associated loci identified in genome-wide association studies in the determination of pediatric BMI. Obesity, 2009, 17 (12): 2254–2257. DOI: 10.1038/oby.2009.159
|
[23] |
van Waas M, Neggers S J C M M, Uitterlinden A G, et al. Treatment factors rather than genetic variation determine metabolic syndrome in childhood cancer survivors. European Journal of Cancer, 2013, 49 (3): 668–675. DOI: 10.1016/j.ejca.2012.09.007
|
[24] |
Locke A E, Kahali B, Berndt S I, et al. Genetic studies of body mass index yield new insights for obesity biology. Nature, 2015, 518 (7538): 197–206. DOI: 10.1038/nature14177
|
[25] |
Mitchell R, Elsworth B L, Mitchell R, et al. MRC IEU UK Biobank GWAS pipeline version 2. 2019. https://doi.org/10.5523/bris.pnoat8cxo0u52p6ynfaekeigi.
|
[26] |
Malik R, Chauhan G, Traylor M, et al. Multiancestry genome-wide association study of 520,000 subjects identifies 32 loci associated with stroke and stroke subtypes. Nature Genetics, 2018, 50 (4): 524–537. DOI: 10.1038/s41588-018-0058-3
|
[27] |
Lyon M S, Andrews S J, Elsworth B, et al. The variant call format provides efficient and robust storage of GWAS summary statistics. Genome Biology, 2021, 22 (1): 32. DOI: 10.1186/s13059-020-02248-0
|
[28] |
Sanderson E, Glymour M M, Holmes M V, et al. Mendelian randomization. Nature Reviews Methods Primers, 2022, 2: 6. DOI: 10.1038/s43586-021-00092-5
|
[29] |
Burgess S, Thompson S G. Use of allele scores as instrumental variables for Mendelian randomization. International Journal of Epidemiology, 2013, 42 (4): 1134–1144. DOI: 10.1093/ije/dyt093
|
[30] |
Pierce B L, Ahsan H, VanderWeele T J. Power and instrument strength requirements for Mendelian randomization studies using multiple genetic variants. International Journal of Epidemiology, 2011, 40 (3): 740–752. DOI: 10.1093/ije/dyq151
|
[31] |
Hu X H, Zhao J, Lin Z X, et al. Mendelian randomization for causal inference accounting for pleiotropy and sample structure using genome-wide summary statistics. Proceedings of the National Academy of Sciences of the United States of America, 2022, 119 (28): e2106858119. DOI: 10.1073/pnas.2106858119
|
Algorithm 1 A bootstrap algorithm for inferring indirect effects |
Require: original sample {\boldsymbol{X}}_1, {\boldsymbol{X}}_2,..., {\boldsymbol{X}}_n |
Ensure: t-test statistic and confidence interval of the indirect effect \beta_M^T\beta_Y^M |
1: compute \hat{\beta}_{M;\gamma_M}^{T}\hat{\beta}_{Y;\gamma_M,\gamma_Y}^{M} using {\boldsymbol{X}}_1, {\boldsymbol{X}}_2,..., {\boldsymbol{X}}_n |
2: for i=1 to B do |
3: generate sample {\boldsymbol{Y}}_1^{i}, {\boldsymbol{Y}}_2^{i},..., {\boldsymbol{Y}}_n^{i} by sampling with replacement from {\boldsymbol{X}}_1, {\boldsymbol{X}}_2,..., {\boldsymbol{X}}_n |
4: compute indirect effect estimate \hat{\beta}_{M;\gamma_M}^{T,(i)}\hat{\beta}_{Y;\gamma_M,\gamma_Y}^{M,(i)} using the sample {\boldsymbol{Y}}_1^{i}, {\boldsymbol{Y}}_2^{i},..., {\boldsymbol{Y}}_n^{i} |
5: end for |
6: compute the standard error \hat{\sigma}_{\beta} of the estimates \hat{\beta}_{M;\gamma_M}^{T,(i)}\hat{\beta}_{Y;\gamma_M,\gamma_Y}^{M,(i)},i=1,2,...,B ; |
7: return Z-test statistic B^{1/2}\hat\beta/\hat\sigma_\beta and confidence interval |
\left(\hat{\beta}_{M;\gamma_M}^{T}\hat{\beta}_{Y;\gamma_M,\gamma_Y}^{M}-B^{-\frac{1}{2}}Z_{\alpha/2}/\hat{\sigma}_{\beta},\ \hat{\beta}_{M;\gamma_M}^{T}\hat{\beta}_{Y;\gamma_M,\gamma_Y}^{M}+B^{-\frac{1}{2}}Z_{\alpha/2}/\hat{\sigma}_{\beta}\right). |
Sample size | (\beta_{M}^T,\beta_{Y}^M) | Our method w/o independence2 | Our method w/ independence3 | Two-step MR | |||||||||||
Bias | SE | SEE | CP | Bias | SE | SEE | CP | Bias | SE | SEE | CP | ||||
No violation ((\gamma_M,\gamma_Y)=(0,0)) | |||||||||||||||
(0.5,0.5) | −0.006 | 0.061 | 0.070 | 0.968 | −0.004 | 0.078 | 0.091 | 0.967 | −0.007 | 0.083 | 0.093 | 0.957 | |||
2000 | −0.004 | 0.042 | 0.046 | 0.956 | −0.002 | 0.057 | 0.061 | 0.945 | −0.005 | 0.055 | 0.062 | 0.953 | |||
0.000 | 0.030 | 0.032 | 0.954 | 0.000 | 0.043 | 0.043 | 0.942 | −0.002 | 0.041 | 0.042 | 0.948 | ||||
(0,0) | −0.002 | 0.009 | 0.019 | 1.000 | 0.000 | 0.014 | 0.028 | 1.000 | 0.000 | 0.013 | 0.026 | 1.000 | |||
2000 | −0.001 | 0.005 | 0.009 | 1.000 | 0.000 | 0.007 | 0.013 | 1.000 | 0.000 | 0.006 | 0.012 | 1.000 | |||
−0.001 | 0.002 | 0.004 | 1.000 | 0.000 | 0.003 | 0.006 | 1.000 | 0.000 | 0.003 | 0.006 | 1.000 | ||||
Mild violation ((\gamma_M,\gamma_Y)=(0.25,0.25)) | |||||||||||||||
(0.5,0.5) | −0.007 | 0.080 | 0.086 | 0.964 | −0.010 | 0.091 | 0.102 | 0.970 | 0.157 | 0.102 | 0.106 | 0.732 | |||
2000 | −0.003 | 0.054 | 0.056 | 0.957 | 0.000 | 0.063 | 0.067 | 0.964 | 0.157 | 0.070 | 0.073 | 0.448 | |||
−0.003 | 0.037 | 0.038 | 0.950 | 0.000 | 0.046 | 0.046 | 0.963 | 0.158 | 0.053 | 0.050 | 0.137 | ||||
(0,0) | −0.003 | 0.015 | 0.025 | 1.000 | −0.001 | 0.017 | 0.033 | 1.000 | 0.013 | 0.029 | 0.034 | 0.997 | |||
2000 | −0.001 | 0.007 | 0.012 | 1.000 | −0.001 | 0.009 | 0.015 | 1.000 | 0.013 | 0.020 | 0.021 | 0.983 | |||
−0.001 | 0.003 | 0.006 | 1.000 | 0.000 | 0.004 | 0.007 | 1.000 | 0.012 | 0.014 | 0.014 | 0.951 | ||||
Strong violation ((\gamma_M,\gamma_Y)=(0.75,0.75)) | |||||||||||||||
(0.5,0.5) | −0.123 | 0.450 | 5.030 | 0.977 | −0.070 | 0.567 | 2.933 | 0.978 | 0.659 | 0.134 | 0.134 | 0.000 | |||
2000 | −0.045 | 0.168 | 0.300 | 0.927 | −0.046 | 0.173 | 0.334 | 0.957 | 0.660 | 0.089 | 0.093 | 0.000 | |||
−0.017 | 0.102 | 0.110 | 0.938 | −0.020 | 0.098 | 0.116 | 0.957 | 0.664 | 0.066 | 0.065 | 0.000 | ||||
(0,0) | −0.031 | 0.078 | 3.006 | 0.999 | −0.049 | 0.200 | 7.663 | 0.999 | 0.225 | 0.077 | 0.085 | 0.283 | |||
2000 | −0.014 | 0.033 | 0.108 | 0.999 | −0.017 | 0.064 | 0.102 | 0.998 | 0.223 | 0.057 | 0.058 | 0.035 | |||
−0.007 | 0.016 | 0.029 | 1.000 | −0.009 | 0.030 | 0.051 | 1.000 | 0.224 | 0.032 | 0.032 | 0.000 | ||||
1 Note: \beta_{M}^T\beta_{Y}^M, true indirect effect; Bias, mean of estimated indirect effects minus true value; SE, standard error of indirect effect estimates; SEE, mean estimated standard errors; CP, coverage probability of confidence interval at level 95\%. 2 Independence assumption between IVs Z_T and Z_M IS NOT imposed on the estimator \hat{\beta}_{M;\gamma_M}^{T}\hat{\beta}_{Y;\gamma_M,\gamma_Y}^{M}. 3 Independence assumption between IVs Z_T and Z_M IS imposed on the estimator \hat{\beta}_{M;\gamma_M}^{T,\perp}\hat{\beta}_{Y;\gamma_M,\gamma_Y}^{M,\perp}. |
Sample size | \beta_{Y}^T | MVMR method | Two-step MR method | |||||||
Bias | SE | SEE | CP | Bias | SE | SEE | CP | |||
No violation ((\beta_T^{Z_M},\beta_M^{Z_T},\beta_Y^{Z_M},\beta_Y^{Z_T})=(0,0,0,0)) | ||||||||||
0.5 | 0.003 | 0.099 | 0.105 | 0.960 | −0.009 | 0.126 | 0.135 | 0.970 | ||
2000 | 0.001 | 0.073 | 0.073 | 0.955 | −0.004 | 0.085 | 0.090 | 0.957 | ||
−0.005 | 0.051 | 0.051 | 0.949 | −0.002 | 0.062 | 0.062 | 0.946 | |||
0 | 0.001 | 0.100 | 0.105 | 0.972 | −0.009 | 0.131 | 0.133 | 0.957 | ||
2000 | 0.000 | 0.073 | 0.073 | 0.955 | −0.005 | 0.091 | 0.090 | 0.952 | ||
−0.001 | 0.051 | 0.051 | 0.955 | −0.002 | 0.063 | 0.062 | 0.943 | |||
Mild violation ((\beta_T^{Z_M},\beta_M^{Z_T},\beta_Y^{Z_M},\beta_Y^{Z_T})=(0.1,0.125,0.2,0.2)) | ||||||||||
0.5 | −0.005 | 0.127 | 0.130 | 0.979 | 0.344 | 0.100 | 0.105 | 0.098 | ||
2000 | 0.000 | 0.085 | 0.088 | 0.973 | 0.343 | 0.072 | 0.071 | 0.005 | ||
0.000 | 0.059 | 0.062 | 0.966 | 0.346 | 0.048 | 0.050 | 0.000 | |||
0 | 0.002 | 0.119 | 0.130 | 0.978 | 0.380 | 0.097 | 0.101 | 0.032 | ||
2000 | 0.004 | 0.086 | 0.089 | 0.960 | 0.376 | 0.069 | 0.070 | 0.001 | ||
−0.003 | 0.063 | 0.062 | 0.950 | 0.376 | 0.047 | 0.048 | 0.000 | |||
Strong violation ((\beta_T^{Z_M},\beta_M^{Z_T},\beta_Y^{Z_M},\beta_Y^{Z_T})=(0.3,0.375,0.6,0.6)) | ||||||||||
0.5 | 0.018 | 0.519 | 5.196 | 1.000 | 0.080 | 0.196 | 0.205 | 0.947 | ||
2000 | −0.003 | 0.277 | 1.283 | 0.997 | 0.095 | 0.139 | 0.139 | 0.885 | ||
0.006 | 0.180 | 0.203 | 0.989 | 0.092 | 0.097 | 0.097 | 0.837 | |||
0 | 0.006 | 0.477 | 4.538 | 0.999 | 0.381 | 0.165 | 0.172 | 0.401 | ||
2000 | −0.008 | 0.265 | 0.993 | 0.996 | 0.380 | 0.117 | 0.116 | 0.110 | ||
−0.003 | 0.188 | 0.204 | 0.985 | 0.372 | 0.081 | 0.081 | 0.004 | |||
\beta_{Y}^T, true direct effect; Bias, mean estimated direct effects minus true value; SE, standard error of indirect effect estimates; SEE, mean estimated standard errors; CP, coverage probability of confidence intervals at level 95\%. |
Sample size | Fully-independent assumption2 | Partially-independent assumption3 | |||||||
Bias | SE | SEE | CP | Bias | SE | SEE | CP | ||
\beta_Y^M=0.5,\beta_M^T=0.5 | |||||||||
0.015 | 0.157 | 0.155 | 0.925 | 0.008 | 0.149 | 0.152 | 0.917 | ||
2000 | 0.005 | 0.106 | 0.102 | 0.928 | 0.009 | 0.102 | 0.103 | 0.934 | |
0.004 | 0.070 | 0.071 | 0.947 | 0.002 | 0.068 | 0.070 | 0.940 | ||
\beta_Y^M=0.5,\beta_M^T=0 | |||||||||
0.006 | 0.074 | 0.073 | 0.984 | 0.003 | 0.074 | 0.073 | 0.989 | ||
2000 | −0.002 | 0.048 | 0.048 | 0.973 | 0.002 | 0.048 | 0.049 | 0.975 | |
0.002 | 0.035 | 0.034 | 0.959 | 0.001 | 0.033 | 0.034 | 0.961 | ||
\beta_Y^M=0,\beta_M^T=0.5 | |||||||||
0.000 | 0.064 | 0.066 | 0.999 | 0.004 | 0.067 | 0.066 | 1.000 | ||
2000 | 0.000 | 0.043 | 0.043 | 0.989 | −0.002 | 0.042 | 0.042 | 0.989 | |
0.000 | 0.028 | 0.029 | 0.976 | 0.000 | 0.029 | 0.029 | 0.978 | ||
\beta_Y^M=0,\beta_M^T=0 | |||||||||
0.001 | 0.015 | 0.020 | 1.000 | 0.000 | 0.016 | 0.020 | 1.000 | ||
2000 | 0.000 | 0.007 | 0.009 | 1.000 | 0.000 | 0.008 | 0.009 | 1.000 | |
0.000 | 0.004 | 0.005 | 1.000 | 0.000 | 0.004 | 0.005 | 1.000 | ||
1 Sample size, size of each subset. \beta_{M}^T\beta_{Y}^M, true indirect effect. Bias, mean estimated indirect effects minus true value; SE, standard error of indirect effect estimates; SEE, mean of estimated standard errors; CP, coverage probability of confidence intervals at level 95\%. 2 The six estimators \hat{\alpha}_{\boldsymbol{A}}^{\boldsymbol{B}}, {\boldsymbol{A}}\in\{{\boldsymbol{T}},{\boldsymbol{M}},{\boldsymbol{Y}}\}, {\boldsymbol{B}}\in\{{\boldsymbol{Z}}_T,{\boldsymbol{Z}}_M\}, are independent. 3 The three estimators (\hat{\alpha}_{{\boldsymbol{T}}}^{{\boldsymbol{Z}}_T},\hat{\alpha}_{{\boldsymbol{T}}}^{{\boldsymbol{Z}}_M}), (\hat{\alpha}_{{\boldsymbol{M}}}^{{\boldsymbol{Z}}_T},\hat{\alpha}_{{\boldsymbol{M}}}^{{\boldsymbol{Z}}_M}), and (\hat{\alpha}_{{\boldsymbol{Y}}}^{{\boldsymbol{Z}}_T},\hat{\alpha}_{{\boldsymbol{Y}}}^{{\boldsymbol{Z}}_M}) are independent. |
Sample size | Fully-independent assumption2 | Partially-independent assumption3 | |||||||
Bias | SE | SEE | CP | Bias | SE | SEE | CP | ||
\beta_Y^T=0.5 | |||||||||
0.002 | 0.196 | 0.199 | 0.969 | −0.001 | 0.190 | 0.193 | 0.958 | ||
2000 | 0.004 | 0.130 | 0.132 | 0.960 | 0.000 | 0.131 | 0.134 | 0.954 | |
−0.001 | 0.093 | 0.094 | 0.959 | −0.001 | 0.092 | 0.094 | 0.954 | ||
\beta_Y^T=0 | |||||||||
−0.005 | 0.133 | 0.138 | 0.956 | −0.004 | 0.132 | 0.137 | 0.960 | ||
2000 | 0.002 | 0.096 | 0.095 | 0.960 | 0.001 | 0.097 | 0.095 | 0.956 | |
−0.003 | 0.067 | 0.067 | 0.960 | −0.001 | 0.065 | 0.066 | 0.955 | ||
\beta_Y^T=-0.5 | |||||||||
−0.002 | 0.141 | 0.137 | 0.958 | −0.006 | 0.138 | 0.137 | 0.954 | ||
2000 | −0.007 | 0.098 | 0.096 | 0.951 | −0.005 | 0.097 | 0.095 | 0.956 | |
−0.002 | 0.067 | 0.067 | 0.947 | −0.002 | 0.065 | 0.066 | 0.953 | ||
1 Sample size, size of each subset; \beta_{Y}^T, true direct effect. Bias, mean estimated indirect effects minus true value; SE, standard error of indirect effect estimates; SEE, mean of estimated standard errors; CP, coverage probability of confidence intervals at level 95\%. 2 The six estimators \hat{\alpha}_{\boldsymbol{A}}^{\boldsymbol{B}}, {\boldsymbol{A}}\in\{{\boldsymbol{T}},{\boldsymbol{M}},{\boldsymbol{Y}}\}, {\boldsymbol{B}}\in\{{\boldsymbol{Z}}_T,{\boldsymbol{Z}}_M\}, are independent. 3 The three estimators (\hat{\alpha}_{{\boldsymbol{T}}}^{{\boldsymbol{Z}}_T},\hat{\alpha}_{{\boldsymbol{T}}}^{{\boldsymbol{Z}}_M}), (\hat{\alpha}_{{\boldsymbol{M}}}^{{\boldsymbol{Z}}_T},\hat{\alpha}_{{\boldsymbol{M}}}^{{\boldsymbol{Z}}_M}), and (\hat{\alpha}_{{\boldsymbol{Y}}}^{{\boldsymbol{Z}}_T},\hat{\alpha}_{{\boldsymbol{Y}}}^{{\boldsymbol{Z}}_M}) are independent. |
Variable | rs2867125 | rs2681492 | |||||
ES | SE | P-val | ES | SE | P-val | ||
BMI | 0.297 | 0.024 | 2.6E−34 | −0.034 | 0.026 | 2.0E−1 | |
SBP | 0.014 | 0.003 | 1.6E−7 | −0.038 | 0.003 | 7.9E−43 | |
Stroke | 0.001 | 0.011 | 9.0E−1 | −0.036 | 0.010 | 2.7E−4 | |
The summary data for the relationship between BMI, SBP, and stroke are taken from three independent GWAS in the IEU OpenGWAS project[27]. ES, genetic effect estimate; SE, standard error of effect estimate; P-val, p-value for significance testing of genetic effect. |