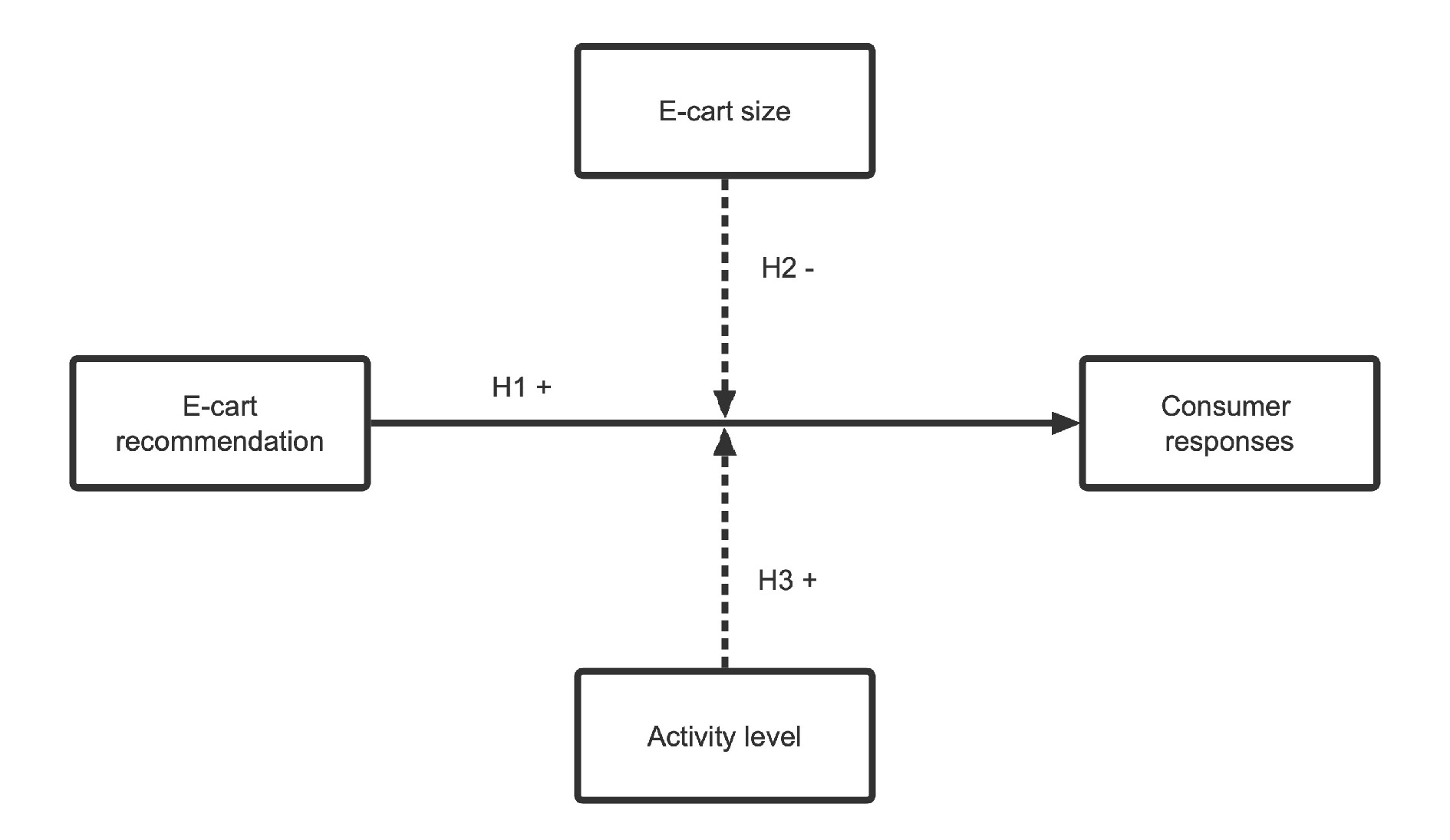
This study aims to compare the effects of e-cart recommendation and homepage recommendation in the field of entertainment products on the basis of a field experiment involving almost 13000 consumers supported by one of the leading digital reading platforms in China. The results indicate that e-cart recommendations have a significant positive impact on consumer downloads in comparison with homepage recommendations. Moreover, this positive effect decreases when the alternatives in the e-cart are of a larger quantity but increases when consumers are more active. Interestingly, this study also finds that e-cart recommendations can spill over to other products, leading to more downloads of non-recommended items. Our findings provide novel insights into consumer responses to e-cart recommendations of entertainment products for researchers and managers alike.
The effects of e-cart recommendation of entertainment products on consumer responses. All hypotheses are supported.
[1] |
Häubl G, Trifts V. Consumer decision making in online shopping environments: The effects of interactive decision aids. Marketing Science, 2000, 19 (1): 4–21. DOI: 10.1287/mksc.19.1.4.15178
|
[2] |
Wang R, Sahin O. The impact of consumer search cost on assortment planning and pricing. Management Science, 2018, 64 (8): 3649–3666. DOI: 10.1287/mnsc.2017.2790
|
[3] |
Virdi P, Kalro A D, Sharma D. Online decision aids: The role of decision-making styles and decision-making stages. International Journal of Retail & Distribution Management, 2020, 48 (6): 555–574. DOI: https://doi.org/10.1108/IJRDM-02-2019-0068
|
[4] |
Lo L Y S, Lin S W, Hsu L Y. Motivation for online impulse buying: A two-factor theory perspective. International Journal of Information Management, 2016, 36 (5): 759–772. DOI: 10.1016/j.ijinfomgt.2016.04.012
|
[5] |
Close A G, Kukar-Kinney M. Beyond buying: Motivations behind consumers’ online shopping cart use. Journal of Business Research, 2010, 63 (9-10): 986–992. DOI: 10.1016/j.jbusres.2009.01.022
|
[6] |
Kapoor A P, Vij M. Following you wherever you go: Mobile shopping “cart-checkout” abandonment. Journal of Retailing and Consumer Services, 2021, 61: 102553. DOI: 10.1016/j.jretconser.2021.102553
|
[7] |
Senecal S, Nantel J. The influence of online product recommendations on consumers’ online choices. Journal of Retailing, 2004, 80 (2): 159–169. DOI: 10.1016/j.jretai.2004.04.001
|
[8] |
Xiao B, Benbasat I. An empirical examination of the influence of biased personalized product recommendations on consumers’ decision making outcomes. Decision Support Systems, 2018, 110: 46–57. DOI: 10.1016/j.dss.2018.03.005
|
[9] |
Lee D, Hosanagar K. How do recommender systems affect sales diversity? A cross-category investigation via randomized field experiment. Information Systems Research, 2019, 30 (1): 239–259. DOI: 10.1287/isre.2018.0800
|
[10] |
Lee D, Gopal A, Park S H. Different but equal? A field experiment on the impact of recommendation systems on mobile and personal computer channels in retail. Information Systems Research, 2020, 31 (3): 892–912. DOI: 10.1287/isre.2020.0922
|
[11] |
Chinchanachokchai S, Thontirawong P, Chinchanachokchai P. A tale of two recommender systems: The moderating role of consumer expertise on artificial intelligence based product recommendations. Journal of Retailing and Consumer Services, 2021, 61: 102528. DOI: 10.1016/j.jretconser.2021.102528
|
[12] |
iResearch. China Internet Entertainment Market Data Release Report 2020Q1&2020Q2e (2020). [2022-08-09]. https://report.iresearch.cn/report_pdf.aspx? id=3603.
|
[13] |
iResearch. Overseas Development of Chinese Network Literature in 2021. 2021. https://report.iresearch.cn/report_pdf.aspx?id=3840
|
[14] |
Shi A, Tan C H, Sia C L. Timing and basis of online product recommendation: The preference inconsistency paradox. In: International Conference on Human Interface and the Management of Information. Berlin, Heidelberg: Springer, 2013: 531–539.
|
[15] |
Yan Q, Zhang L, Li Y, et al. Effects of product portfolios and recommendation timing in the efficiency of personalized recommendation. Journal of Consumer Behavior, 2016, 15 (6): 516–526. DOI: 10.1002/cb.1588
|
[16] |
Hennig-Thurau T, Houston M B. Entertainment Science. Cham, Switzerland: Springer, 2019.
|
[17] |
Foutz N Z. Entertainment Marketing (Foundations and Trends® in Marketing). Boston: Now Publishers Inc, 2017.
|
[18] |
Dhar R, Wertenbroch K. Consumer choice between hedonic and utilitarian goods. Journal of Marketing Research, 2000, 37 (1): 60–71. DOI: 10.1509/jmkr.37.1.60.18718
|
[19] |
Lee D, Hosanagar K. How do product attributes and reviews moderate the impact of recommender systems through purchase stages? Management Science, 2020, 67 (1): 524–546. DOI: 10.1287/mnsc.2019.3546
|
[20] |
Okada E M. Justification effects on consumer choice of hedonic and utilitarian goods. Journal of Marketing Research, 2005, 42 (1): 43–53. DOI: 10.1509/jmkr.42.1.43.56889
|
[21] |
Clement M, Fabel S, Schmidt-Stolting C. Diffusion of hedonic goods: A literature review. The International Journal on Media Management, 2006, 8 (4): 155–163. DOI: 10.1207/s14241250ijmm0804_1
|
[22] |
Aggarwal P, Vaidyanathan R. Perceived effectiveness of recommendation agent routines: Search vs. experience goods. International Journal of Internet Marketing and Advertising, 2005, 2 (1): 38–55. DOI: 10.1504/IJIMA.2005.007503
|
[23] |
Fitzsimons G J, Lehmann D R. Reactance to recommendations: When unsolicited advice yields contrary responses. Marketing Science, 2004, 23 (1): 82–94. DOI: 10.1287/mksc.1030.0033
|
[24] |
Wang J, Zhang Y. Opportunity model for e-commerce recommendation: Right product; right time. In: Proceedings of the 36th International ACM SIGIR Conference on Research and Development in Information Retrieval. New York: ACM, 2013: 303–312.
|
[25] |
Todri V, Ghose A, Singh P V. Trade-offs in online advertising: Advertising effectiveness and annoyance dynamics across the purchase funnel. Information Systems Research, 2019, 31 (1): 102–125. DOI: 10.1287/isre.2019.0877
|
[26] |
Campbell M C, Keller K L. Brand familiarity and advertising repetition effects. Journal of Consumer Research, 2003, 30 (2): 292–304. DOI: 10.1086/376800
|
[27] |
Toubia O, Iyengar G, Bunnell R, et al. Extracting features of entertainment products: A guided latent dirichlet allocation approach informed by the psychology of media consumption. Journal of Marketing Research, 2019, 56 (1): 18–36. DOI: 10.1177/0022243718820559
|
[28] |
Platania M, Platania S, Santisi G. Entertainment marketing, experiential consumption and consumer behavior: The determinant of choice of wine in the store. Wine Economics and Policy, 2016, 5 (2): 87–95. DOI: 10.1016/j.wep.2016.10.001
|
[29] |
Setyani V, Zhu Y Q, Hidayanto A N, et al. Exploring the psychological mechanisms from personalized advertisements to urge to buy impulsively on social media. International Journal of Information Management, 2019, 48: 96–107. DOI: 10.1016/j.ijinfomgt.2019.01.007
|
[30] |
Longoni C, Cian L. Artificial intelligence in utilitarian vs. hedonic contexts: The “word-of-machine” effect. Journal of Marketing, 2022, 86 (1): 91–108. DOI: 10.1177/0022242920957347
|
[31] |
Botti S, McGill A L. The locus of choice: Personal causality and satisfaction with hedonic and utilitarian decisions. Journal of Consumer Research, 2011, 37 (6): 1065–1078. DOI: 10.1086/656570
|
[32] |
Sinha S K, Verma P. Impact of sales promotion’s benefits on perceived value: Does product category moderate the results? Journal of Retailing and Consumer Services, 2020, 52: 101887. DOI: 10.1016/j.jretconser.2019.101887
|
[33] |
Parra J F, Ruiz S. Consideration sets in online shopping environments: The effects of search tool and information load. Electronic Commerce Research and Applications, 2009, 8 (5): 252–262. DOI: 10.1016/j.elerap.2009.04.005
|
[34] |
Ghiassaleh A, Kocher B, Czellar S. Best seller!? Unintended negative consequences of popularity signs on consumer choice behavior. International Journal of Research in Marketing, 2020, 37 (4): 805–820. DOI: 10.1016/j.ijresmar.2020.04.003
|
[35] |
Wang J, Sarwar B, Sundaresan N. Utilizing related products for postpurchase recommendation in e-commerce. In: Proceedings of the Fifth ACM Conference on Recommender Systems. New York: ACM, 2011: 329–332.
|
[36] |
Lee L, Ariely D. Shopping goals, goal concreteness, and conditional promotions. Journal of Consumer Research, 2006, 33 (1): 60–70. DOI: 10.1086/504136
|
[37] |
Kwon K, Cho J, Park Y. Influences of customer preference development on the effectiveness of recommendation strategies. Electronic Commerce Research and Applications, 2009, 8 (5): 263–275. DOI: 10.1016/j.elerap.2009.04.004
|
[38] |
Song T, Yi C, Huang J. Whose recommendations do you follow? An investigation of tie strength, shopping stage, and deal scarcity. Information & Management, 2017, 54 (8): 1072–1083. DOI: 10.1016/j.im.2017.03.003
|
[39] |
Schreiner T, Rese A, Baier D. Multichannel personalization: Identifying consumer preferences for product recommendations in advertisements across different media channels. Journal of Retailing and Consumer Services, 2019, 48: 87–99. DOI: 10.1016/j.jretconser.2019.02.010
|
[40] |
Luo X, Lu X, Li J. When and how to leverage e-commerce cart targeting: The relative and moderated effects of scarcity and price incentives with a two-stage field experiment and causal forest optimization. Information Systems Research, 2019, 30 (4): 1203–1227. DOI: 10.1287/isre.2019.0859
|
[41] |
Tsao W Y. The fitness of product information: Evidence from online recommendations. International Journal of Information Management, 2013, 33 (1): 1–9. DOI: 10.1016/j.ijinfomgt.2012.04.003
|
[42] |
Dai Q, Cui X L. The influence and moderating effect of trust in streamers in a live streaming shopping environment. JUSTC, 2022, 52 (2): 6. DOI: 10.52396/JUSTC-2021-0219
|
[43] |
Hauser J R, Wernerfelt B. An evaluation cost model of consideration sets. Journal of consumer research, 1990, 16 (4): 393–408. DOI: 10.1086/209225
|
[44] |
Iyengar S S, Lepper M R. When choice is demotivating: Can one desire too much of a good thing? Journal of Personality and Social Psychology, 2000, 79 (6): 995–1006. DOI: 10.1037/0022-3514.79.6.995
|
[45] |
Kuksov D, Villas-Boas J M. When more alternatives lead to less choice. Marketing Science, 2010, 29 (3): 507–524. DOI: 10.1287/mksc.1090.0535
|
[46] |
Mittal B. The maximizing consumer wants even more choices: How consumers cope with the marketplace of overchoice. Journal of Retailing and Consumer Services, 2016, 100 (31): 361–370. DOI: 10.1016/j.jretconser.2016.05.003
|
[47] |
Choudhary V, Currim I, Dewan S, et al. Evaluation set size and purchase: Evidence from a product search engine. Journal of Interactive Marketing, 2017, 37: 16–31. DOI: 10.1016/j.intmar.2016.07.003
|
[48] |
Zhu F, Zhang X. Impact of online consumer reviews on sales: The moderating role of product and consumer characteristics. Journal of Marketing, 2010, 74 (2): 133–148. DOI: 10.1509/jm.74.2.133
|
[49] |
Clement J, Aastrup J, Forsberg S C. Decisive visual saliency and consumers’ in-store decisions. Journal of Retailing and Consumer Services, 2015, 22: 187–194. DOI: 10.1016/j.jretconser.2014.09.002
|
[50] |
Helmers C, Krishnan P, Patnam M. Attention and saliency on the internet: Evidence from an online recommendation system. Journal of Economic Behavior & Organization, 2019, 161: 216–242. DOI: https://doi.org/10.1016/j.jebo.2019.04.010
|
[51] |
Zhu D H, Wang Y W, Chang Y P. The influence of online cross-recommendation on consumers’ instant cross-buying intention: The moderating role of decision-making difficulty. Internet Research, 2018, 28 (3): 604–622. DOI: 10.1108/IntR-05-2017-0211
|
[52] |
Lleras J S, Masatlioglu Y, Nakajima D, et al. When more is less: Limited consideration. Journal of Economic Theory, 2017, 170: 70–85. DOI: 10.1016/j.jet.2017.04.004
|
[53] |
Hong W, Thong J Y, Tam K Y. How do web users respond to nonbanner-ads animation? The effects of task type and user experience. Journal of the American Society for Information Science and Technology, 2007, 58 (10): 1467–1482. DOI: 10.1002/asi.20624
|
[54] |
Resnick M, Albert W. The impact of advertising location and user task on the emergence of banner ad blindness: An eye-tracking study. International Journal of Human-Computer Interaction, 2014, 30 (3): 206–219. DOI: 10.1080/10447318.2013.847762
|
[55] |
Darley W K, Blankson C, Luethge D J. Toward an integrated framework for online consumer behavior and decision making process: A review. Psychology & Marketing, 2010, 27 (2): 94–116. DOI: https://doi.org/10.1002/mar.20322
|
[56] |
Li L R, Luo B, Sun Y, et al. Research on the influence mechanism of green advertising on consumers’ intention to purchase energy-saving products: Based on the SOR model. JUSTC, 2021. DOI: 10.52396/JUSTC-2021-0015
|
[57] |
Zhang K D, Fang W P, Luo B, et al. New product launching: The effect of firm-generated content on purchase intention. JUSTC, 2021, 51 (12): 912–926. DOI: 10.52396/JUST-2021-0107
|
[58] |
Gai P J, Klesse A K. Making recommendations more effective through framings: Impacts of user- versus item-based framings on recommendation click-throughs. Journal of Marketing, 2019, 83 (6): 61–75. DOI: 10.1177/0022242919873901
|
[59] |
Knuth M, Behe B K, Hall C R, et al. Sit back or dig in: The role of activity level in landscape market segmentation. HortScience, 2019, 54 (10): 1818–1823. DOI: 10.21273/HORTSCI14158-19
|
[60] |
Ren X, Cao J, Xu X, et al. A two-stage model for forecasting consumers’ intention to purchase with e-coupons. Journal of Retailing and Consumer Services, 2021, 59: 102289. DOI: 10.1016/j.jretconser.2020.102289
|
[61] |
Aydinli A, Bertini M, Lambrecht A. Price promotion for emotional impact. Journal of Marketing, 2014, 78 (4): 80–96. DOI: 10.1509/jm.12.0338
|
[62] |
Karmarkar U R, Shiv B, Knutson B. Cost conscious? The neural and behavioral impact of price primacy on decision making. Journal of Marketing Research, 2015, 52 (4): 467–481. DOI: 10.1509/jmr.13.0488
|
[63] |
Walia N, Srite M, Huddleston W. Eyeing the web interface: The influence of price, product, and personal involvement. Electronic Commerce Research, 2016, 16 (3): 297–333. DOI: 10.1007/s10660-015-9200-9
|
[64] |
Jiang Y, Shang J, Liu Y, et al. Redesigning promotion strategy for e-commerce competitiveness through pricing and recommendation. International Journal of Production Economics, 2015, 167: 257–270. DOI: 10.1016/j.ijpe.2015.02.028
|
[65] |
Kawaguchi K, Uetake K, Watanabe Y. Effectiveness of product recommendations under time and crowd pressures. Marketing Science, 2019, 38 (2): 253–273. DOI: 10.1287/mksc.2018.1132
|
[66] |
Li J, Luo X, Lu X, et al. The double-edged effects of e-commerce cart retargeting: Does retargeting too early backfire? Journal of Marketing, 2021, 85 (4): 123–140. DOI: 10.1177/0022242920959043
|
Variable name | Variable description | Mean | SD | Min | Max |
Download | Dummy to indicate whether a consumer downloaded the recommended novel within one month after the recommendation campaign; Download = 1 for downloaded, and 0 otherwise. | 0.60 | 0.49 | 0 | 1 |
Treat | Dummy to indicate whether a consumer is in treatment group or control group; Treat = 1 for treatment group, and 0 otherwise. | 0.50 | 0.50 | 0 | 1 |
E-cart size | Number of novels in consumer’s e-cart. | 3 | 5.40 | 0 | 140 |
Activity level | Individual activity level of a consumer. | 5.47 | 10.82 | 0 | 279 |
Pre_download chapters | Number of chapters a consumer downloaded within one month before the activity. | 231.10 | 952.26 | 0 | 26724 |
Pre_download days | Number of days a consumer downloaded within one month before the activity. | 3.49 | 5.33 | 0 | 31 |
Pre_pay novels | Number of novels a consumer paid for within one month before the activity. | 0.48 | 1.20 | 0 | 18 |
Pre_all pay | Total amount paid by a consumer within one month before the activity. | 2.36 | 10.06 | 0 | 268.51 |
Pre_pay days | Number of days a consumer paid within one month before the activity. | 1.42 | 4.22 | 0 | 31 |
Gender | Dummy to indicate gender; Gender = 1 for female, and 0 otherwise. | 0.40 | 0.49 | 0 | 1 |
Nickname | Dummy to indicate whether an account has a nickname; Nickname = 1 for having a nickname, and 0 otherwise. | 0.30 | 0.46 | 0 | 1 |
Phone type | Dummy to indicate mobile phone type; Phone type = 1 for iPhone, and 0 otherwise. | 0.25 | 0.43 | 0 | 1 |
Registration duration | Length of consumer registration (unit: day). | 442.40 | 361.43 | 1 | 2449 |
Category | Dummy to indicate novel category; Category =1 for female channel, and 0 for male channel. | 0.52 | 0.50 | 0 | 1 |
Update | Dummy to indicate novel update status; Update = 1 for being updated, and 0 otherwise. | 0.93 | 0.25 | 0 | 1 |
Novel chapters | Total number of chapters of a novel. | 2403 | 1341.91 | 604 | 4805 |
Novel words | Total number of words of a novel. | 5356973 | 3005694 | 1458526 | 12047318 |
Heat | Number of consumers recommending a novel. | 229.50 | 316.46 | 0 | 1509 |
Observations | Pre_download chapters | Pre_download days | Activity level | Pre_pay novels | Pre_all pay | Pre_pay days | |
Treatment group | 6377 | 233.08 | 3.45 | 5.45 | 0.48 | 2.27 | 1.40 |
Control group | 6495 | 229.12 | 3.53 | 5.49 | 0.47 | 2.45 | 1.43 |
p value | N/A | 0.81 | 0.37 | 0.85 | 0.49 | 0.31 | 0.71 |
Dependent variable | Download Logit (1) | Download Logit (2) |
Treat | 3.27 *** | 3.29 *** |
(0.05) | (0.05) | |
Constant | −0.90 *** | −1.04 *** |
(0.03) | (0.10) | |
Consumer | ||
Gender | 0.23 *** | |
(0.06) | ||
Nickname | 0.10 | |
(0.06) | ||
Phone type | −0.06 | |
(0.07) | ||
Registration duration | −0.02 | |
(0.03) | ||
Novel | ||
Category | 0.17 * | |
(0.08) | ||
Update | −0.06 | |
(0.09) | ||
Novel chapters | −0.18 | |
(0.16) | ||
Novel words | 0.22 | |
(0.15) | ||
Heat | −0.05 | |
(0.04) | ||
R2 | 0.41 | 0.42 |
Observations | 12872 | 12872 |
p < 0.1; *p < 0.05; **p <0.01; ***p <0.001. |
Dependent variable | Download logit (1) | Download chapters Tobit (2) | Download chapters Tobit (3) |
Treat | 0.22 * | 187.30 *** | 189.00 *** |
(0.09) | (4.77) | (4.81) | |
Constant | −0.05 | −178.40 *** | −184.60 *** |
(0.08) | (3.90) | (10.07) | |
Consumer | Yes | No | Yes |
Novel | Yes | No | Yes |
R2 | 0.02 | 0.02 | 0.02 |
Observations | 12872 | 12872 | 12872 |
p < 0.1; *p < 0.05; **p <0.01; ***p <0.001. |
Dependent variable | Download Logit (1) | Download chapters Tobit (2) |
Treat×(E-cart size) | −0.48 *** | −30.16 *** |
(0.06) | (3.81) | |
Treat×(Activity level) | 0.11 | 26.41 *** |
(0.07) | (3.22) | |
Treat | 3.32 *** | 189.20 *** |
(0.05) | (4.81) | |
E-cart size | 0.31 *** | 23.07 *** |
(0.06) | (5.34) | |
Activity level | −0.01 | 0.92 |
(0.06) | (3.47) | |
Constant | −1.04 *** | −184.00 *** |
(0.10) | (10.04) | |
Consumer | Yes | Yes |
Novel | Yes | Yes |
R2 | 0.42 | 0.02 |
Observations | 12872 | 12872 |
p < 0.1; *p < 0.05; **p <0.01; ***p <0.001. |
Dependent variable | Other download Logit (1) | All download Logit (2) | Other download chapters Tobit (3) | All download chapters Tobit (4) |
Treat | 1.51 *** | 2.96 *** | 54.49 | 102.20 *** |
(0.06) | (0.11) | (30.15) | (29.96) | |
Constant | 2.48 *** | 2.94 *** | 278.20 *** | 283.80 *** |
(0.16) | (0.19) | (64.77) | (64.60) | |
Consumer | Yes | Yes | Yes | Yes |
Novel | Yes | Yes | Yes | Yes |
R2 | 0.18 | 0.26 | 0.02 | 0.02 |
Observations | 12872 | 12872 | 12872 | 12872 |
p < 0.1; *p < 0.05; **p <0.01; ***p <0.001. |