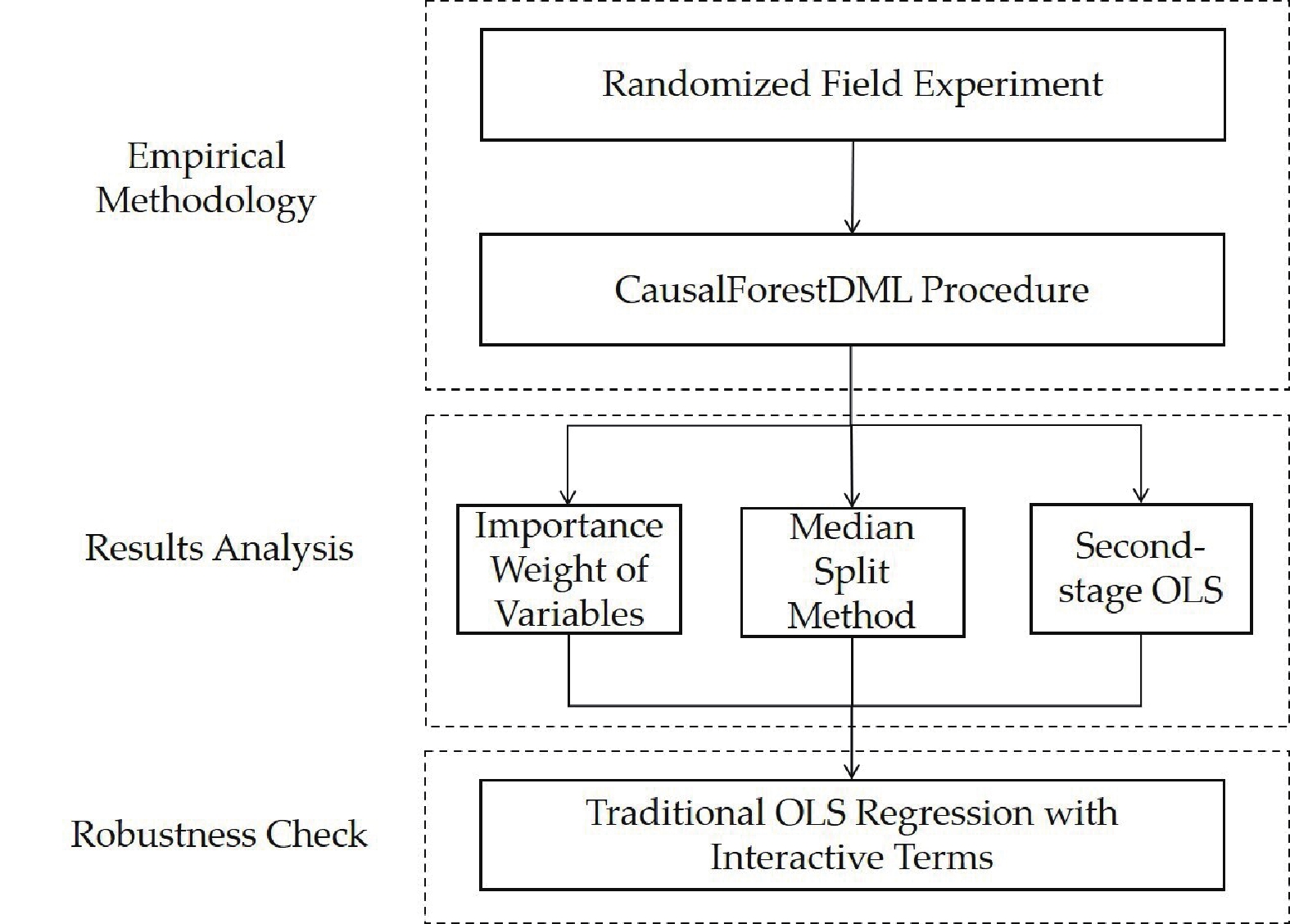
Many digital platforms have employed free-content promotion strategies to deal with the high uncertainty levels regarding digital content products. However, the diversity of digital content products and user heterogeneity in content preference may blur the impact of platform promotions across users and products. Therefore, free-content promotion strategies should be adapted to allocate marketing resources optimally and increase revenue. This study develops personalized free-content promotion strategies based on individual-level heterogeneous treatment effects and explores the causes of their heterogeneity, focusing on the moderating effect of user engagement-related variables. To this end, we utilize random field experimental data provided by a top Chinese e-book platform. We employ a framework that combines machine learning with econometric causal inference methods to estimate individual treatment effects and analyze their potential mechanisms. The analysis shows that, on average, free-content promotions lead to a significant increase in consumer payments. However, the higher the level of user engagement, the lower the payment lift caused by promotions, as more-engaged users are more strongly affected by the cannibalization effect of free-content promotion. This study introduces a novel causal research design to help platforms improve their marketing strategies.
The hypothesis test result of this study.
[1] |
Choi A A, Cho D, Yim D, et al. When seeing helps believing: The interactive effects of previews and reviews on e-book purchases. Information Systems Research, 2019, 30 (4): 1164–1183. DOI: 10.1287/isre.2019.0857
|
[2] |
Ghose A, Han S P. Estimating demand for mobile applications in the new economy. Management Science, 2014, 60 (6): 1470–1488. DOI: 10.1287/mnsc.2014.1945
|
[3] |
Hoang A-P, Kauffman R J. Content sampling, household informedness, and the consumption of digital information goods. Journal of Management Information Systems, 2018, 35 (2): 575– 609. DOI: 10.1080/07421222.2018.1451958
|
[4] |
Ascarza E. Retention futility: Targeting high-risk customers might be ineffective. Journal of Marketing Research, 2018, 55 (1): 80–98. DOI: 10.1509/jmr.16.0163
|
[5] |
Fudenberg D, Villas-Boas J M. Behavior-based price discrimination and customer recognition. In: Handbook on Economics and Information Systems, Volume 1. Amsterdam: Elsevier, 2006 : 377–436.
|
[6] |
Ellickson P B, Kar W, Reeder J C. Estimating marketing component effects: Double machine learning from targeted digital promotions. Marketing Science, 2022, 42 (4): 704–728. DOI: 10.1287/mksc.2022.1401
|
[7] |
Gopalakrishnan A, Park Y-H. The impact of coupons on the visit-to-purchase funnel. Marketing Science, 2021, 40 (1): 48–61. DOI: 10.1287/mksc.2020.1232
|
[8] |
Sahni N S, Zou D, Chintagunta P K. Do targeted discount offers serve as advertising? Evidence from 70 field experiments. Management Science, 2017, 63 (8): 2688–2705. DOI: 10.1287/mnsc.2016.2450
|
[9] |
Zhang Y, Li B, Luo X, et al. Personalized mobile targeting with user engagement stages: Combining a structural hidden Markov model and field experiment. Information Systems Research, 2019, 30 (3): 787–804. DOI: 10.1287/isre.2018.0831
|
[10] |
Bawa K, Shoemaker R. The effects of free sample promotions on incremental brand sales. Marketing Science, 2004, 23 (3): 345–363. DOI: 10.1287/mksc.1030.0052
|
[11] |
Ho Y-J, Dewan S, Ho Y-C. Distance and local competition in mobile geofencing. Information Systems Research, 2020, 31 (4): 1421– 1442. DOI: 10.1287/isre.2020.0953
|
[12] |
Sahni N S, Wheeler S C, Chintagunta P. Personalization in email marketing: The role of noninformative advertising content. Marketing Science, 2018, 37 (2): 236–258. DOI: 10.1287/mksc.2017.1066
|
[13] |
Ghose A, Li B, Liu S. Mobile targeting using customer trajectory patterns. Management Science, 2019, 65 (11): 5027–5049. DOI: 10.1287/mnsc.2018.3188
|
[14] |
Bonfrer A, Drèze X. Real-time evaluation of e-mail campaign performance. Marketing Science, 2009, 28 (2): 251–263. DOI: 10.1287/mksc.1080.0393
|
[15] |
Venkatesan R, Farris P W. Measuring and managing returns from retailer-customized coupon campaigns. Journal of Marketing, 2012, 76 (1): 76–94. DOI: 10.1509/jm.10.0162
|
[16] |
Lemmens A, Croux C. Bagging and boosting classification trees to predict churn. Journal of Marketing Research, 2006, 43 (2): 276–286. DOI: 10.1509/jmkr.43.2.276
|
[17] |
Risselada H, Verhoef P C, Bijmolt T H. Staying power of churn prediction models. Journal of Interactive Marketing, 2010, 24 (3): 198–208. DOI: 10.1016/j.intmar.2010.04.002
|
[18] |
Hung S-Y, Yen D C, Wang H-Y. Applying data mining to telecom churn management. Expert Systems with Applications, 2006, 31 (3): 515–524. DOI: 10.1016/j.eswa.2005.09.080
|
[19] |
Wager S, Athey S. Estimation and inference of heterogeneous treatment effects using random forests. Journal of the American Statistical Association, 2018, 113 (523): 1228–1242. DOI: 10.1080/01621459.2017.1319839
|
[20] |
Hitsch G J, Misra S. Heterogeneous treatment effects and optimal targeting policy evaluation. SSRN 3111957, 2018 .
|
[21] |
Sutcliffe A. Designing for User Engagement: Aesthetic and Attractive User Interfaces. Cham, Switzerland: Springer, 2009.
|
[22] |
Kim Y H, Kim D J, Wachter K. A study of mobile user engagement (MoEN): Engagement motivations, perceived value, satisfaction, and continued engagement intention. Decision Support Systems, 2013, 56: 361–370. DOI: 10.1016/j.dss.2013.07.002
|
[23] |
O’Brien H L, Toms E G. What is user engagement? A conceptual framework for defining user engagement with technology. Journal of the American Society for Information Science and Technology, 2008, 59 (6): 938–955. DOI: 10.1002/asi.20801
|
[24] |
O’Brien H, Cairns P. Why Engagement Matters: Cross-Disciplinary Perspectives of User Engagement in Digital Media. Cham, Switzerland: Springer, 2016 .
|
[25] |
Gu Z, Bapna R, Chan J, et al. Measuring the impact of crowdsourcing features on mobile app user engagement and retention: A randomized field experiment. Management Science, 2022, 68 (2): 1297–1329. DOI: 10.1287/mnsc.2020.3943
|
[26] |
Van Doorn J, Lemon K N, Mittal V, et al. Customer engagement behavior: Theoretical foundations and research directions. Journal of Service Research, 2010, 13 (3): 253–266. DOI: 10.1177/1094670510375599
|
[27] |
Ahuja V, Medury Y. Corporate blogs as e-CRM tools — Building consumer engagement through content management. Journal of Database Marketing & Customer Strategy Management, 2010, 17 (2): 91–105. DOI: 10.1057/dbm.2010.8
|
[28] |
Lee D, Hosanagar K, Nair H S. Advertising content and consumer engagement on social media: Evidence from Facebook. Management Science, 2018, 64 (11): 5105–5131. DOI: 10.1287/mnsc.2017.2902
|
[29] |
Hong Y, Pavlou P A. Product fit uncertainty in online markets: Nature, effects, and antecedents. Information Systems Research, 2014, 25 (2): 328–344. DOI: 10.1287/isre.2014.0520
|
[30] |
Pavlou P A, Liang H, Xue Y. Understanding and mitigating uncertainty in online exchange relationships: A principal-agent perspective. MIS Quarterly, 2007, 31 (1): 105–136. DOI: 10.2307/25148783
|
[31] |
Dimoka A, Hong Y, Pavlou P A. On product uncertainty in online markets: Theory and evidence. MIS Quarterly, 2012, 36 (2): 395– 426. DOI: 10.2307/41703461
|
[32] |
Matt C, Hess T. Product fit uncertainty and its effects on vendor choice: An experimental study. Electronic Markets, 2016, 26 (1): 83–93. DOI: 10.1007/s12525-015-0199-5
|
[33] |
Nelson P. Information and consumer behavior. Journal of Political Economy, 1970, 78 (2): 311–329. DOI: 10.1086/259630
|
[34] |
Lin Z, Zhang Y, Tan Y. An empirical study of free product sampling and rating bias. Information Systems Research, 2019, 30 (1): 260–275. DOI: 10.1287/isre.2018.0801
|
[35] |
Chen P, Hitt L M, Hong Y, et al. Measuring product type and purchase uncertainty with online product ratings: a theoretical model and empirical application. Information Systems Research, 2021, 32 (4): 1470–1489. DOI: 10.1287/isre.2021.1041
|
[36] |
Godes D. Product policy in markets with word-of-mouth communication. Management Science, 2017, 63 (1): 267–278. DOI: 10.1287/mnsc.2015.2330
|
[37] |
Marks L J, Kamins M A. The use of product sampling and advertising: Effects of sequence of exposure and degree of advertising claim exaggeration on consumers’ belief strength, belief confidence, and attitudes. Journal of Marketing Research, 1988, 25 (3): 266–281. DOI: 10.2307/3172529
|
[38] |
Foubert B, Gijsbrechts E. Try it, you’ll like it—or will you? The perils of early free-trial promotions for high-tech service adoption. Marketing Science, 2016, 35 (5): 810–826. DOI: 10.1287/mksc.2015.0973
|
[39] |
Halbheer D, Stahl F, Koenigsberg O, et al. Choosing a digital content strategy: How much should be free. International Journal of Research in Marketing, 2014, 31 (2): 192–206. DOI: 10.1016/j.ijresmar.2013.10.004
|
[40] |
Godinho de Matos M, Ferreira P. The effect of binge-watching on the subscription of video on demand: Results from randomized experiments. Information Systems Research, 2020, 31 (4): 1337– 1360. DOI: 10.1287/isre.2020.0948
|
[41] |
Chernozhukov V, Chetverikov D, Demirer M, et al. Double/debiased machine learning for treatment and structural parameters. The Econometrics Journal, 2018, 21 (1): C1–C68. DOI: 10.1111/ectj.12097
|
[42] |
Rubin D B. Estimating causal effects of treatments in randomized and nonrandomized studies. Journal of Educational Psychology, 1974, 66 (5): 688–701. DOI: 10.1037/h0037350
|
[43] |
Athey S, Tibshirani J, Wager S. Generalized random forests. The Annals of Statistics, 2019, 47 (2): 1148–1178. DOI: 10.1214/18-AOS1709
|
[44] |
Robins J M, Rotnitzky A, Zhao L P. Estimation of regression coefficients when some regressors are not always observed. Journal of the American Statistical Association, 1994, 89 (427): 846–866. DOI: 10.1080/01621459.1994.10476818
|
[45] |
Luo X, Lu X, Li J. When and how to leverage e-commerce cart targeting: The relative and moderated effects of scarcity and price incentives with a two-stage field experiment and causal forest optimization. Information Systems Research, 2019, 30 (4): 1203– 1227. DOI: 10.1287/isre.2019.0859
|
[46] |
Guo T, Sriram S, Manchanda P. The effect of information disclosure on industry payments to physicians. Journal of Marketing Research, 2021, 58 (1): 115–140. DOI: 10.1177/0022243720972106
|
[47] |
Cong Z, Liu J, Manchanda P. The role of ‘live’ in livestreaming markets: Evidence using orthogonal random forest. SSRN 3878605, 2021 .
|
[48] |
Kruglanski A W, Higgins E T. Social Psychology: Handbook of Basic Principles. New York: The Guilford Press, 2013 .
|
[49] |
Reza S, Ho H, Ling R, et al. Experience effect in the impact of free trial promotions. Management Science, 2021, 67 (3): 1648–1669. DOI: 10.1287/mnsc.2020.3613
|
[50] |
Reimers I, Xie C. Do coupons expand or cannibalize revenue? Evidence from an e-market. Management Science, 2019, 65 (1): 286–300. DOI: 10.1287/mnsc.2017.2934
|
Variable | Description |
Prepromotion user characteristics | |
PrePay | Paid amount of the user in the month prior to the experiment (CNY) |
PreRead | Reading time of the user in the month prior to the experiment (min) |
PreLogin | Number of user logins in the month prior to the experiment |
Tenure | Registration time of the user (d) |
Pushed book characteristics | |
ChapterCount | Number of chapters in the book |
Updays | Time the book has been on shelf (d) |
GoodComments | Number of positive reviews |
BadComments | Number of negative reviews |
Vip | A binary variable indicating whether it is a book that VIP users can purchase at a discount (Yes: 1, No: 0) |
Postpromotion user payment | |
Y | Amount paid by the user for the pushed book in the promotion month (CNY) |
Variable | Mean | Std | Min | Max |
Prepromotion user characteristics | ||||
PrePay | 0.878 | 7.317 | 0 | 103.140 |
PreRead | 14.439 | 99.000 | 0 | |
PreLogin | 25.401 | 62.686 | 0 | 507 |
Tenure | 108.596 | 321.556 | 10 | |
Pushed book characteristics | ||||
ChapterCount | 48.766 | 128 | 465 | |
Updays | 654.128 | 551.447 | 4 | |
GoodComments | 573.256 | 1733.386 | 0 | |
BadComments | 60.267 | 143.505 | 0 | |
Vip | 0.374 | 0.484 | 0 | 1 |
Postpromotion user payment | ||||
Y(CNY) | 6.344 | 8.752 | 0 | 154.620 |
Control | Treatment | p-values | ||||
Mean | Std | Mean | Std | |||
Number of participants | 3576 | 3576 | ||||
PrePay | 0.907 | 7.152 | 0.849 | 7.480 | 0.737 | |
PreRead | 16.352 | 89.089 | 12.526 | 107.985 | 0.102 | |
PreLogin | 25.812 | 68.653 | 24.990 | 56.094 | 0.580 | |
Tenure | 102.872 | 327.093 | 114.321 | 315.865 | 0.132 |
Variable | Importance | Rank |
Prepromotion user data | ||
PrePay | 8.973% | 4 |
PreRead | 10.837% | 3 |
PreLogin | 42.861% | 1 |
Tenure | 6.875% | 6 |
Pushed book feature | ||
ChapterCount | 14.530% | 2 |
Updays | 8.387% | 5 |
GoodComments | 3.730% | 7 |
BadComments | 3.408% | 8 |
Vip | 0.400% | 9 |
Payment lift | N | Mean | Std | [95% conf. interval] | |
ˆτPrePay=0 | 6850 | 9.647 | 1.585 | 7.244 | 12.050 |
ˆτPrePay>0 | 302 | 4.465 | 3.168 | −1.015 | 9.945 |
ˆτPreRead=0 | 5915 | 9.864 | 1.436 | 7.497 | 12.231 |
ˆτPreRead>0 | 1237 | 7.343 | 2.744 | 3.805 | 10.881 |
ˆτPreLogin≤1 | 3798 | 10.346 | 0.937 | 8.318 | 12.373 |
ˆτPreLogin>1 | 3354 | 8.389 | 2.308 | 5.252 | 11.526 |
ˆτ | 7152 | 9.428 | 1.979 | 6.821 | 12.036 |
Variable | ˆτid | |
Coeff. | SE | |
Prepromotion user data | ||
LogPrePay | −0.722*** | (0.031) |
LogPreRead | −0.282*** | (0.014) |
LogPreLogin | −0.746*** | (0.012) |
LogTenure | −0.129*** | (0.019) |
Pushed book feature | ||
ChapterCount | 0.003*** | (0.000) |
Updays | − |
(0.000) |
GoodComments | 0.000 | (0.000) |
BadComments | 0.042 | (0.033) |
VIP | 11.024*** | (0.100) |
R2 | 0.587 | |
Observations | 7152 | |
*** p<0.01, ** p<0.05, * p<0.1. Values in parentheses are standard errors. |
Variable | Yid | |
Coeff. | SE | |
Treatment | 10.817*** | (0.235) |
Treatment interacted with Subclass | ||
PrePay>0 | −2.632*** | (0.888) |
PreRead>0 | −4.141*** | (0.538) |
PreLogin>1 | −0.945** | (0.365) |
Intercept | 0.855*** | (0.370) |
Control variables | Included | |
R2 | 0.387 | |
Observations | 7152 | |
*** p<0.01, ** p<0.05, * p<0.1. Values in parentheses are standard errors. |